AI Powered Data Analysis
In today’s fast-paced business environment, organisations rely on sales data to make informed decisions and stay ahead of the competition. However, manually analysing vast amounts of data can be time-consuming and prone to errors.
Leveraging Generative AI (GenAI) and Large Language Models (LLMs), businesses can transform their sales analysis processes, unlocking valuable insights that drive revenue growth and operational efficiency.
This case study explores how an AI agent can revolutionise sales data analysis through advanced analytics, automation, and predictive capabilities.
The little pop-up blue square in the corner is an AI Agent that has been given some sample sales data, please feel free to click on it and ask it some questions.
Business Challenge
Addressing Specific Business Problems with GenAI
An AI agent can help your company with the following struggles:
-
Data Overload: Large volumes of sales data are often underutilised due to the complexity of manual analysis.
-
Inconsistent Decision-Making: Sales teams rely on intuition rather than data-driven insights.
-
Missed Revenue Opportunities: Inefficient pricing strategies, lack of cross-selling insights, and poor demand forecasting lead to lost sales.
-
Operational Bottlenecks: Sales reporting and analysis require extensive manual effort, reducing productivity.
-
Competitive Pressures: Businesses lack real-time insights to benchmark performance and anticipate market shifts.
AI Solution Implemented
Internal Platforms, RAG, and Optimisation
Why not just throw all your sales data excel spreadsheets into a secure AI ‘knowledge base’ and get it to do the heavy lifting for you. Our demo agent agent has the following abilities:
-
Generative AI and LLM Integration: The AI agent uses LLMs to process and interpret complex sales data, providing actionable insights.
-
Retrieval-Augmented Generation (RAG): The AI retrieves and contextualises relevant sales reports, market trends, and historical data to enhance decision-making.
-
Automated Dashboards and Reports: AI-driven analytics generate real-time visualisations, allowing businesses to track sales performance effortlessly.
-
Predictive Sales Forecasting: Machine learning models anticipate demand fluctuations, optimising pricing and inventory management.
-
CRM and ERP Integration: Seamless data connectivity with existing business systems ensures smooth operations and workflow automation.
Implementation Process
Enhancing Efficiency and Productivity
Our Agent was deployed through a structured implementation plan:
-
Data Integration: The AI agent was connected to sales databases, CRM, and ERP systems.
-
Model Training & Fine-Tuning: Using historical sales data to improve forecasting accuracy, machine learning models were trained.
-
Dashboard & Reporting Automation: AI-generated reports and interactive dashboards replaced manual spreadsheets.
-
User Adoption & Training: Sales teams were trained to interpret AI insights and apply recommendations.
-
Continuous Monitoring & Optimisation: The AI system was continuously refined based on real-time feedback and evolving business needs.
Click below to see how our AI Agent actually works
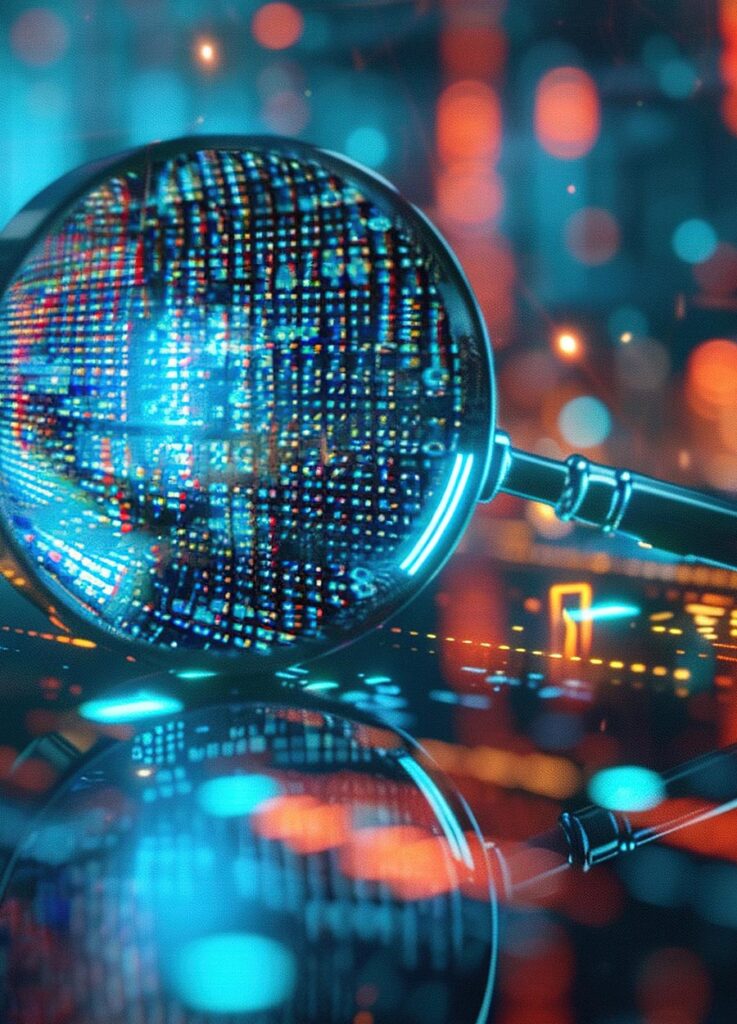
Results & Business Impact
AI-Driven Transformation
The implementation of our AI-powered sales analysis agent delivered significant improvements:
-
Enhanced Decision-Making: AI identified key sales trends, enabling proactive strategies.
-
Revenue Growth: AI-driven pricing optimisation and cross-sell recommendations led to a 15% increase in sales.
-
Improved Customer Targeting: Personalised marketing campaigns resulted in a 20% boost in customer engagement.
-
Operational Efficiency: Automated reporting saved an estimated 500+ hours of manual analysis per year.
-
Optimised Inventory Management: Demand forecasting reduced stock outs by 30% and minimised overstocking.
-
Competitive Advantage: AI-driven benchmarking provided real-time insights into market trends and competitor performance.
Challenges, Lessons Learned, and Future Plans
Despite the success, the project encountered some challenges:
-
Data Quality Issues: Initial inconsistencies in sales data required data cleansing efforts.
-
User Resistance: Some sales teams were hesitant to trust AI-driven insights.
-
Integration Complexities: Customising AI workflows to fit existing systems required additional development time.
Lessons learned include the importance of user training, continuous model improvement, and seamless system integration. Moving forward, the company plans to enhance the AI solution by:
-
Expanding AI capabilities to include natural language queries for intuitive data analysis.
-
Integrating external market intelligence to improve sales forecasting.
-
Scaling AI adoption across multiple business units for holistic decision-making.
Conclusion
AI as a Competitive Advantage
By leveraging Generative AI and LLMs, businesses can transform sales data analysis into a strategic asset that drives efficiency, revenue growth, and customer engagement. The AI-powered sales analysis agent improves decision-making and empowers sales teams with real-time insights, automation, and predictive analytics. As AI continues to evolve, organisations that embrace AI-driven sales analytics will gain a sustainable competitive edge in the market.
We have more stories here here
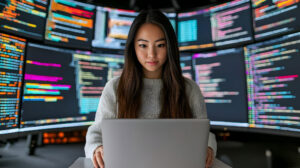
AI Isn’t Just Tech – It’s a Strategic Ally
AI helps businesses process huge volumes of data, identifying patterns and forecasting future outcomes.
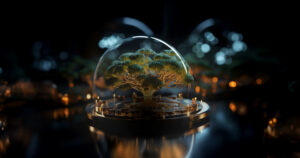
Powerful AI, Zero Data Risk: Secure, Private, and In-House
In an era where AI is revolutionising industries, many companies face a critical dilemma: how to leverage the power of AI without compromising data sovereignty.
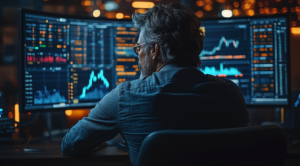
AI Powered Data Analysis
In today’s fast-paced business environment, organisations rely on sales data to make informed decisions and stay ahead of the competition.